Artificial Intelligence: An Accelerating Revolution
November 20, 2024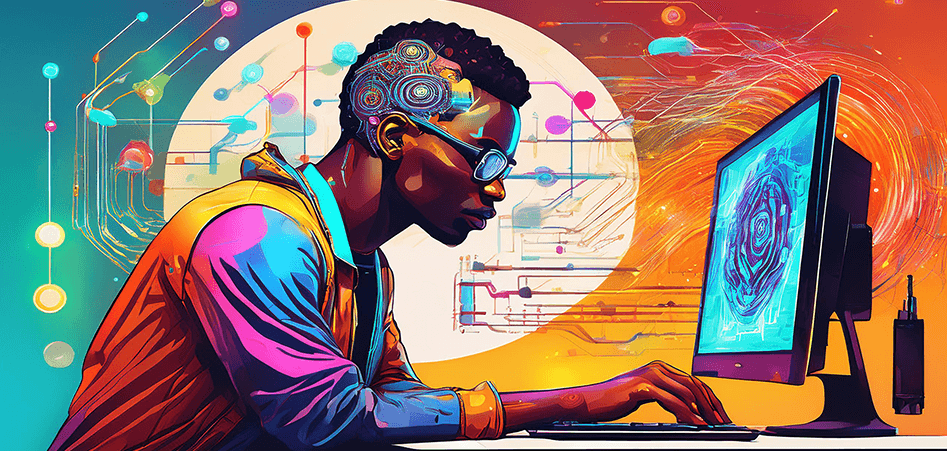
This article was written by several investment professionals at Jennison Associates as of October 2024.
In 2023, Jennison, a long-standing partner at Harbor, wrote that we are in the opening stages of an Artificial Intelligence (AI)-driven investment cycle that has the potential to transform the technology sector and, ultimately, the global economy (Exhibit 1). Today, the revolutionary impact of AI-driven change is becoming evident in most industries and is accelerating.
Below, Jennison details how AI demand is growing for technology hardware, new capabilities of AI-related application products, and the challenges (and some surprises) that have arisen from AI. Overall, we believe AI represents an extraordinary opportunity, and investors can benefit from an understanding of the current AI ecosystem as well as the full implications of its future development.
Exhibit 1: The AI Wave Is Sweeping Through the Economy
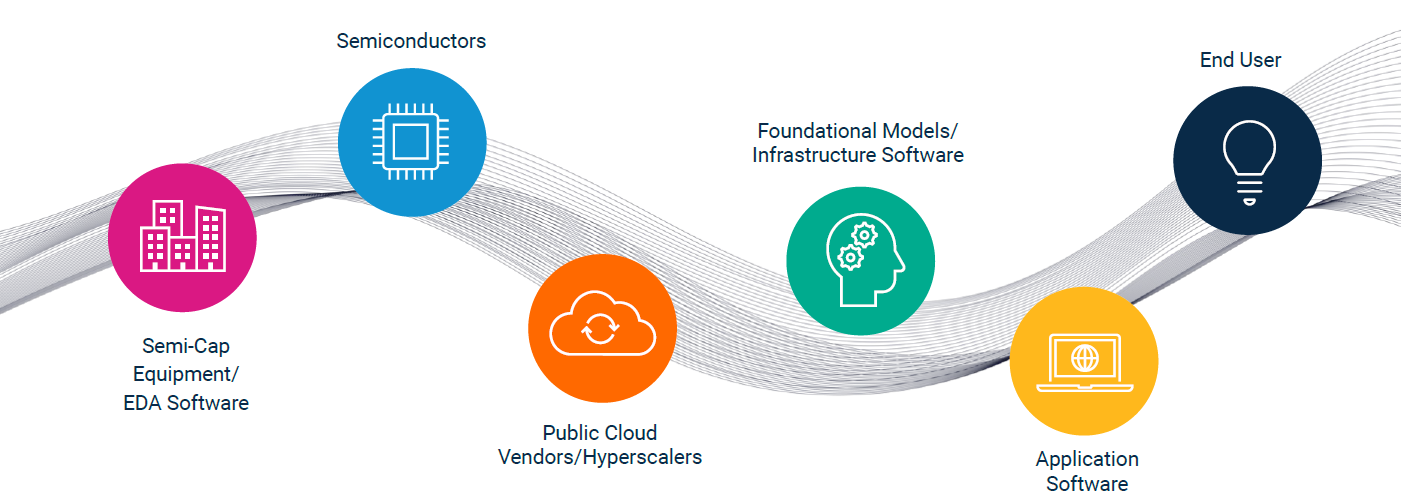
The information is provided for illustrative and educational purposes only and should not be considered investment advice.
Source: Jennison
A Rising Tide of Demand
One of the earliest and most tangible impacts of AI has been surging demand for the semiconductors and infrastructure needed to support AI model training and running scaled AI-enabled applications. (Nvidia—the leading producer of graphic processing units [GPUs]—has been among the largest and most publicly recognized beneficiaries of this trend.) A notable source of this demand has come from large hyperscale cloud computing companies, which continue to be the major buyers of AI hardware (e.g., GPUs and application-specific integrated circuits, or ASICs). Capital expenditures and spending forecasts for many company platforms— including Microsoft’s Azure, Amazon Web Services, Google, Oracle, and Meta—have risen significantly (Exhibit 2).
Exhibit 2: Cloud CapEx and Expectations Have Risen
Capital Expenditures (US dollars in millions)
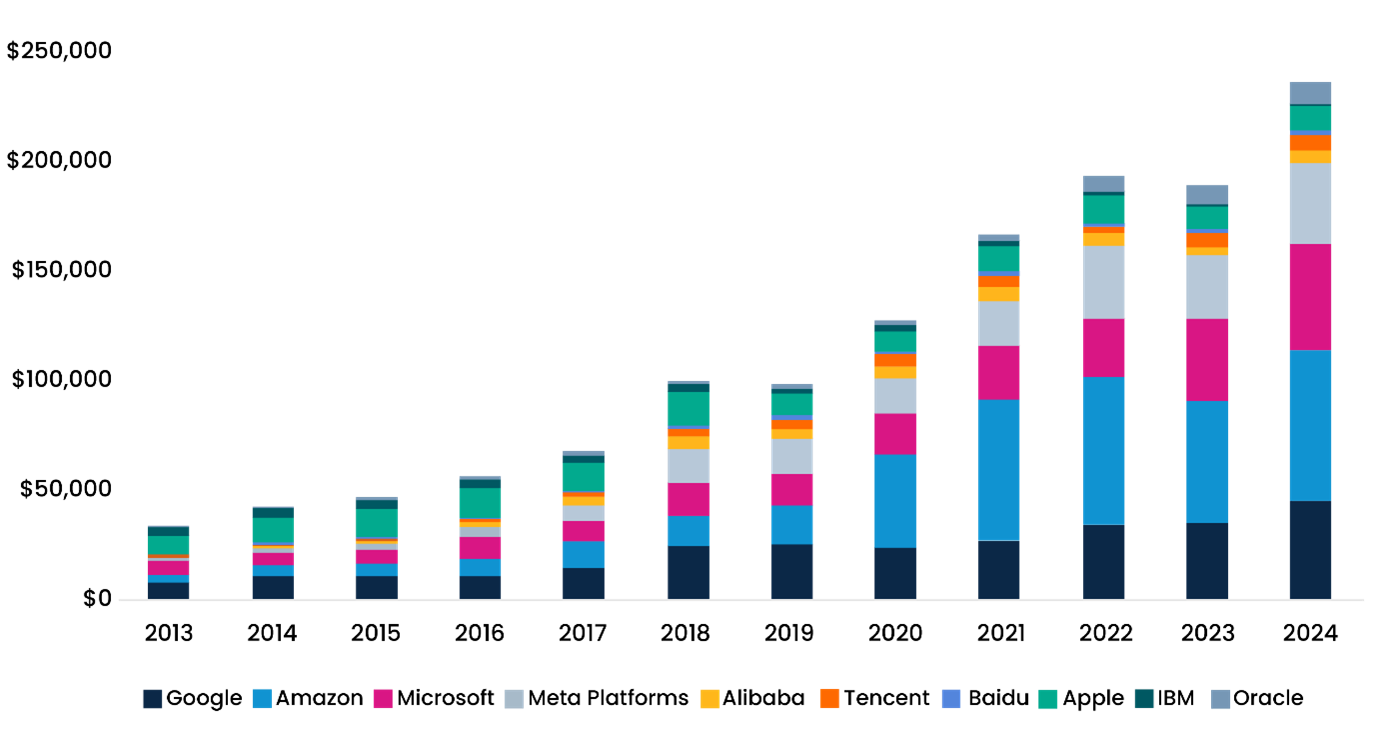
2023 and 2024 figures are estimated.
Source: Morgan Stanley
These companies plan to rent this hardware to customers of all sizes, including software firms, to train models and run production AI applications. As demand for these services has grown, the companies have continued to build out the scale required to bring products to market. We believe this is a critical part of the “AI wave” that is preceding the creation and adoption of the products by end users.
Given the extraordinary levels of demand, it is normal for many competitors to enter the market and, over time, challenge the incumbents. In mid-2024, however, we have yet to observe this dynamic. In fact, while there have been new entrants in the semiconductor space, these competitors have been able to take advantage of the growing market without having any meaningful negative impact on the strength of the established, dominant companies.
National AI Capabilities
One source of demand for AI capabilities has come from sovereign funds, which have sought AI expertise to build models in their own languages. This acknowledges the tremendous potential influence and reach of AI technology, as governments have decided that AI is too important a cultural and social force to be left to the markets alone.
As the market broadens for accelerated computing, demand has risen for other chips, such as ASICs, which are custom products designed for smaller or more specific workloads. In addition, AI users have sought ways to improve their network efficiency, which has raised demand for ethernet technology.
Energy Demand Brings Opportunities
Dramatic change rarely occurs without some challenges. In AI, we are closely watching rising energy usage (Exhibit 3). Training a single AI model could emit as much as 5x the lifetime emissions of an average car1, and the energy needed to run GPUs is already taxing local power grids in states such as Virginia.2 This has created a demand for companies that can boost efficiencies in the AI process. Higher energy usage is also impacting the design of hardware. Semiconductor firms are looking to make their chips run faster and more efficiently, and several opportunities have appeared in lesser-known areas, such as companies that develop chip-cooling applications.
Exhibit 3: Demand for Power for Datacenters Is Forecasted to Rise Significantly in the US
US datacenter energy consumption, Terawatt hour (TWh)
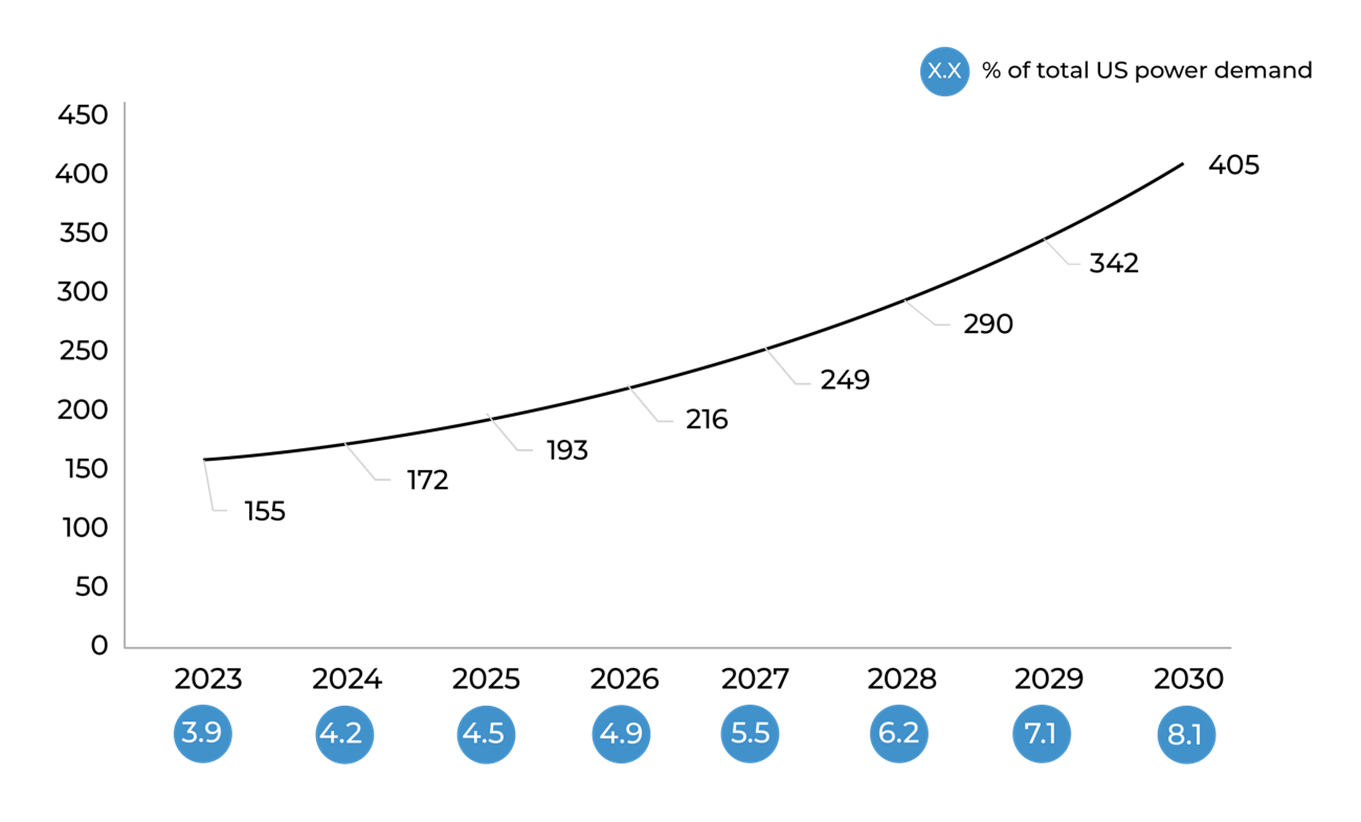
Source: McKinsey Energy Solutions Global Energy Perspective 2023; McKinsey datacenter demand model
Companies are starting to disseminate power usage by pushing computing out of data centers and back into the terminal. This is a notable exception to the trend of having computing power centralized in the cloud. It is also another example of how AI is transforming the entire semiconductor industry—not just the most sophisticated, best-positioned players—and leading to a new Supercycle of demand for semiconductors with advanced packaging.
Practical Potential: Use Cases
While AI has had a measurable impact on technology hardware, its influence on the rest of technology—and the economy—has been much more fluid and has been unfolding in real time. It is important to note that AI did not suddenly appear when ChatGPT was released in November 2022. AI-related development has been a priority at many companies for several years. These companies have developed expertise in AI, which is now being used to develop and test specific use cases in generative AI.
Software and Applications
Software applications that use generative AI have been introduced, and firms are employing these new tools in their workflows. As a result, software developers are gathering data and user feedback in real time, which is being used to make the software offerings more effective. As these new generations of AI-powered products come online, we are looking for evidence of improved processes and efficiencies for their customers. Over time, we believe this should result in higher productivity and stronger economic growth.
Some of the earliest examples of AI-driven improvements have been developed by software firms dedicated to customer service, marketing, and sales. Several firms reported that a sizable portion of their enterprise customers are already using AI features, and they expect their AI capabilities will improve significantly within a year. These AI tools are initially targeting routine tasks that can be automated, accelerated, or enhanced. For example, AI-related software can draft emails (ranging in style from formal to casual) and combine them with AI-generated images for use in a marketing campaign. The campaign may also include meeting follow-ups and automated scheduling. Internally, the software tracks and analyzes data, offering a transparent, real-time view of a company’s sales pipeline and the effectiveness of its sales efforts.
Advertising
Generative AI is helping advertisers create content, which we believe will especially benefit advertising on social media platforms. Several large online advertising companies, such as Meta, are testing tools that can automatically resize an image or video and convert still images into videos—e.g., a picture of a runner can become a short video of that runner in a featured pair of shoes—and generate a range of content with relatively little effort. With these tools, AI-generated content can be altered quickly and easily to fit into several different media formats. This increases the amount of content that the recommendation engine can show the end user and can result in better outcomes for both them and the advertiser.
Over time, we expect more advertisers to use AI video generation tools like OpenAI’s Sora, Google’s Veo, (or similar technology) for creative objectives. Today, it can be expensive to create a video advertisement for a medium-sized business. We believe AI tools will cut production costs dramatically over time, which will lower the barrier to entry for small- and medium-sized businesses to adopt video advertising. Social media platforms and the largest video hosting services should be the primary beneficiaries of this development.
Cybersecurity
Another area for widespread AI use is cybersecurity, where firms are developing AI tools to help companies keep their networks and data secure. Much of this is designed to help a firm’s tech security identify a specific threat, uncover where it could exploit the firm’s network, and implement remediation. These tools are being marketed as stand-alone products that can free up security and data teams to do more important tasks—a critical need due to a massive shortage of cybersecurity professionals and growing threats from cybercriminals.
Agents
AI agents are a new development framework that may be the next frontier in the use of large language models (LLMs). An AI agent is software designed to perform complex tasks autonomously or semi-autonomously. AI agents can create action plans, reason, determine the need for external actions or tools, gather additional information from the user in a conversational format, interact with other AI agents, use tools to interact with external systems (websites, software APIs), revise its plan based on its research, and proactively start a series of actions.
AI agents are empowered and have access to tools, but they do require oversight and direction. Having software that is akin to a virtual employee that can plan, reason, learn, and act based on the data it creates and retrieves creates new possibilities. Unlike its non-agent counterpart, an AI agent can act on a high-level prompt—such as, “I would like to book a trip to Paris in May with my family”—through a series of logical steps and the use of external tools (e.g., a web browser). This technology is still relatively nascent and available at just a few companies. However, these systems have the potential to massively broaden the use of AI systems, which could impact a variety of industries.
By comparison, non-agentic systems attempt to execute complex tasks in one attempt. For example, if a non-agentic system writes an essay, it generates the text, but it does not reread it and reflect on what it said. By contrast, an AI agent might approach the task of writing an essay by creating a comprehensive plan and breaking the task into several logical steps: create an outline; research related topics through the internet or a knowledge base; write a draft; review and ask itself a series of clarifying or critical questions about content and structure; do further research on those questions; generate a final essay (Exhibit 4).
Exhibit 4: Agent of Progress: AI Agent Capabilities vs. Non-AI Agent
Aspect | Non-AI Agent System | AI Agent System |
Understanding the Prompt | Might identify keywords such as "book," "trip," "Paris," "May," but may not fully grasp family travel intent. | Understands natural language, recognizes the goal of planning a family trip, and potentially infers preferences based on past searches or user profile (if available). |
Initial Actions | May offer a list of travel booking websites or a search engine prompt for "Paris travel". | Begins breaking down the task into sub-goals: finding flights, hotels suitable for families, Paris activities in May, etc. |
Scope of Assistance | Likely focused on individual components (e.g., flights, lodging, meals) requiring the user to manage most aspects independently. | Aims to provide a more comprehensive solution by suggesting itineraries, child-friendly attractions, and even consider visa requirements or packing tips. |
Personalization | Limited personalization based on past bookings or basic preferences. | Uses past travel history, location data, or explicit inquiries to create a tailored experience (e.g., suggesting kid-friendly hotels or restaurants, offering activities based on indicated interests). |
Proactivity | Might require prompting each step to find flights, hotels, etc. | Proactively searches for deals, compares flights across various dates, might suggest travel insurance, or check for family friendly room options. |
Handling Ambiguity | Many struggle if the user does not provide specific budget, exact dates, or the number of family members. | Engages in a conversation to clarify missing details, asks questions such as "What's your budget?" or "How many children are traveling?" |
For illustrative purposes only.
Source: Jennison
While this process can take longer (and cost more), the results can be significantly improved. For tasks that require a high-quality response, agents could unlock more serious enterprise use cases for customer-facing applications. More advanced systems will likely have several specialized agents working together to improve the results of their work.
An AI agent can broaden the range of use cases for LLMs, allowing them to take on more complex multi-step tasks and take actions in external systems to achieve specific goals. Agents should help reduce hallucinations and allow the systems to take actions, thus expanding the range of tasks that an AI system can execute.
What Comes Next? Challenges and Opportunities
As with software, companies are encountering challenges as they seek to exploit the benefits of AI tools. Most companies outside of technology understand the importance of AI, but they have little experience or expertise in the infrastructure needed to build out their AI capabilities. Making it more difficult, the collection of software programs (or the “software stack”) supporting the AI features is new and always improving. Another major challenge is data purity. As companies (especially small- and medium-sized enterprises) look to build internal AI applications, their proprietary data may be scattered, stored inefficiently, or inaccessible.
As a result, we think most companies will likely consume their first generative AI software products through new and existing Service as a Software (SaaS) vendors. These SaaS vendors have the expertise to build AI products quickly and integrate them into existing products (and customer workflows). They also have their data in order, and they have economies of scale (build once and sell many times). Furthermore, SaaS companies that can make it easy for their customers to utilize their proprietary data will have a competitive advantage. We believe this will drive demand for SaaS products with the technical resources to address these needs.
Over time, we see more non-technology companies using their proprietary data to build their own internal applications. These applications could drive meaningful competitive and business achievements, but they will likely require more time to build and put into production. We also note that competition for talented workers is fierce, especially from giant, well-resourced tech companies.
We also believe the falling cost of running AI applications will help to catalyze the move from R&D into production. Pricing for LLMs and multimodal models fell dramatically in 2023 because of improvements in model efficiency and the scaling up of GPU infrastructure from cloud providers. Use cases that were previously very expensive to run at a production scale are now much more affordable.
Overall, the appearance of AI-driven tools has been uneven. While most companies have incorporated AI into their strategic plans, they are in different stages of development—some companies have years of AI experience and have introduced AI-driven products, while others are just beginning to understand the implications of AI.
An industry where everyone appears to be winning may seem like a boon for investors, but we believe extra vigilance is warranted. The AI story is well known, and money has poured into investment opportunities. We expect that winners—and losers—will emerge. If individual companies stumble—e.g., through poor execution, management uncertainty, or bad publicity—the decline could be swift and result in significant losses.
Investors seem to be, despite mid-summer volatility, patient. However, we believe that clarity will start to emerge in 2025. Many technology companies will showcase their AI products at user conferences and in other public settings, and we will closely monitor the customer reaction to the new offerings.
The AI Revolution Is No Hallucination
It has been less than two years since the launch of Chat-GPT changed the public perception of AI’s capabilities. We continue to believe that AI represents a revolutionary technology that could ultimately transform the global economy. Over the past several months, we have seen evidence that we underestimated the speed and scope of AI-related change.
In hardware, demand for AI has boosted the prospects of incumbents, their competitors, and ancillary technologies that support the AI ecosystem. In software applications, as in hardware, AI tools are being rolled out in a number of industries, where we expect they can drive sales and increase productivity. We are mindful that AI faces a number of challenges, which we are watching closely.
Above all, the environment remains fluid and uncertain as the technology continues to change at an accelerating pace. Definitive, widely accepted AI applications within the enterprise have not appeared, but promising uses of AI are already evident among early adopters. It remains a process of trial and error, and we expect many failures. However, we are convinced that “AI haves and have-nots” will appear, with those companies that have successfully embraced AI in stronger competitive positions versus their slower or less skilled peers. Ultimately, we are looking for the companies that get it right—that develop “killer use cases” and build effective AI infrastructure and apps that lead to monetization.
In our view, these represent attractive long-term opportunities, and we believe that investors with the resources, experience, expertise, and disciplined approach are best positioned to exploit them.
Important Information
Endnotes
1 Karen Hao, “Training a single AI model can emit as much carbon as five cars in their lifetimes,” MIT Technology Review, June 6, 2019.
2 Carly Davenport, et al., “AI, data centers and the coming US power demand surge,” Goldman Sachs Equity Research, April 28, 2024, p. 8.
AI hallucination is a phenomenon wherein a large language model (LLM)—often a generative AI chatbot or computer version tool—perceives patterns or objects that are nonexistent or imperceptible to human observers, creating outputs that are nonsensical or altogether inaccurate.
The opinions expressed are as of October 2024 and are subject to change. The opinions expressed by the investment professional authors at Jennison and do not necessarily represent the views of Harbor Capital Advisors, Inc.
This information has been provided by Jennison Associates for informational purposes only. It does not constitute or form part of any offer to issue or sell, or any solicitation of any offer to subscribe or to purchase, shares, units or other interests in investments that may be referred to herein and must not be construed as investment or financial product advice.
References to specific company securities are for illustration only and should not be construed as an offer or solicitation from Harbor Capital to buy or sell any securities.
Harbor nor Jennison has not considered any reader’s financial situation, objective or needs in providing the relevant information.
Investing entails risks and there can be no assurance that any investment will achieve profits or avoid incurring losses.
Stock markets are volatile and equity values can decline significantly in response to adverse issuer, political, regulatory, market and economic conditions. Past performance is not necessarily a guide to future performance or returns.
Jennison has taken all reasonable care to ensure that the information contained in this material is accurate at the time of its distribution, no representation or warranty, express or implied, is made as to the accuracy, reliability or completeness of such information.
This material may contain forward-looking information that is not purely historical in nature. Such information may include, among other things, projections and forecasts. There is no guarantee that any of these views will come to pass.
Harbor Capital Advisors is not affiliated with Jennison Associates.
Copyright © (2024) Harbor Capital Advisors, Inc. All Rights Reserved.
4040133